The evolution of deepfake technology, which is constantly becoming more advanced and affordable, is forcing businesses to seek more effective tools to address this growing threat.
Surprisingly, the most challenging part for fraudsters using deepfakes to bypass online identity verification (IDV) lies not in generating but in presenting them to such systems. Does this mean that companies can rely on well-established IDV tools for deepfake detection?
In this blog post, we’ll explore the vulnerabilities in IDV systems targeted by deepfakes, and highlight deepfake detection strategies to prevent such attacks effectively.
How deepfakes can exploit the weaknesses of IDV systems
The online identity verification process, where a user’s selfie is typically presented, can be simplified into three key steps: data capture, analysis, and evaluation—resulting in a “verified” or “not verified” decision.
Depending on the scenario—whether identifying a new user or authenticating a returning one— the analysis stage may involve comparing the presented identifier against a database of known individuals or performing specific authenticity checks, such as verifying the selfie against the portrait in the user’s ID document.
In all cases, fraudsters aim to exploit weak points in IDV systems. This applies to both “classic” tools, such as silicone masks or mannequins, and modern techniques like deepfakes. Importantly, defenders know about these weak points too.
The ISO/IEC 30107 standard on biometric presentation attack detection identifies at least nine vulnerable stages in the selfie verification process where attacks could occur. Wrapping up these findings with the key verification stages, we arrive at the following map of potential attack points:
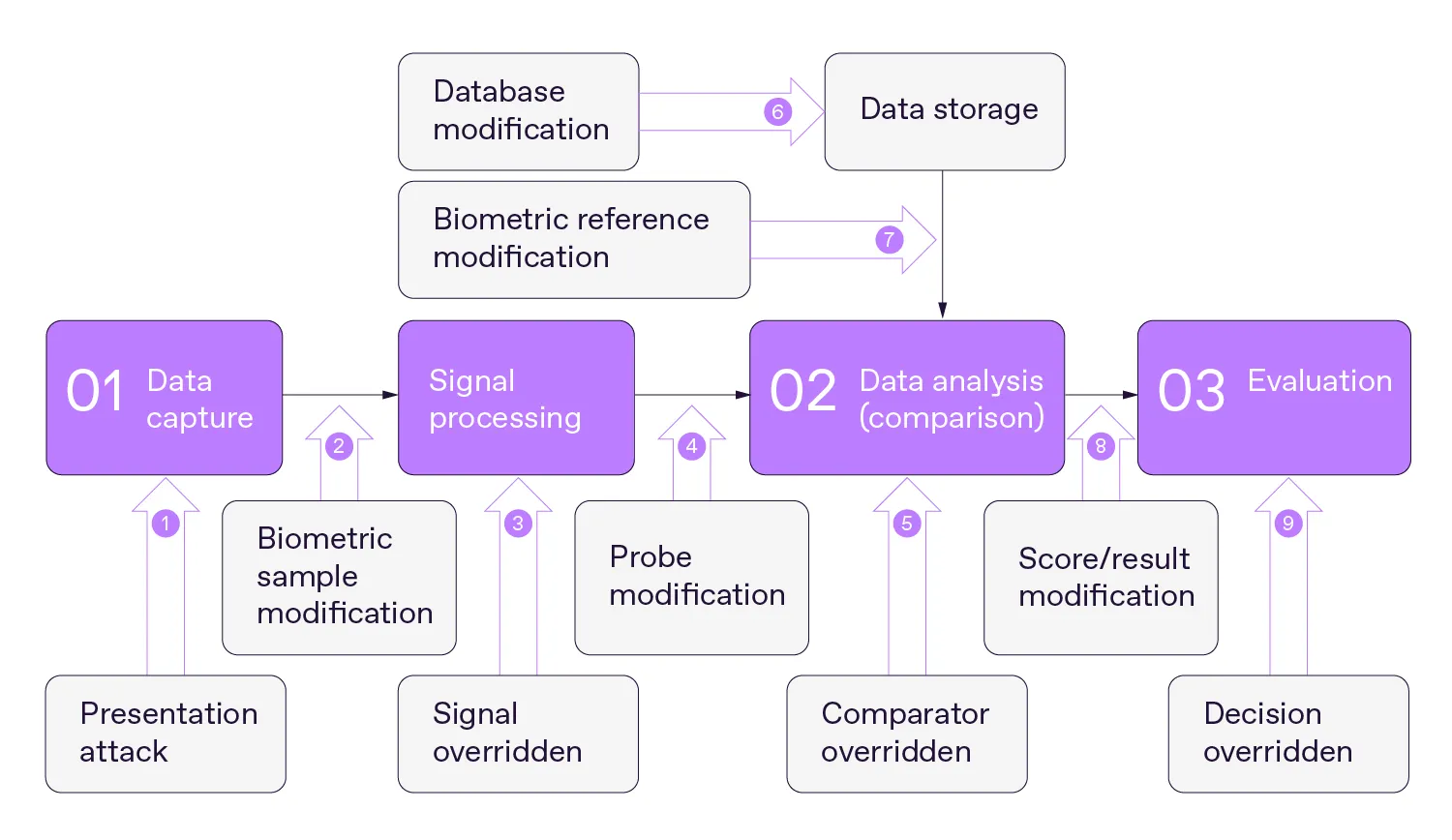
According to ISO/IEC 30107, there are at least nine vulnerable points in biometric systems that fraudsters can target.
With this idea in mind, the question is: where can deepfakes be exploited? In practice, there are two main ways to utilize deepfakes when attacking biometric systems:
Present fake images or videos to the biometric system without interacting with the entire process (Point 1 in the diagram)
Inject deepfake data into the flow (Points 2, 4, and 7).
Simply put, deepfakes can be used for presentation or injection attacks. Now let’s see where the difference is between these methods.
When deepfakes are presented to the system
Fraudsters traditionally use various tools for face presentation attacks, such as printed photos, paper cutouts, multi-layered or silicon masks, 3D prints, mannequins, and photo or video editing software like Photoshop. This mimics legitimate users but relies on physical or hybrid methods.
Deepfakes, being 100% digital, are less adaptable to physical forms. The most effective method to deploy them is as an on-screen image or video presented directly to the camera.
When deepfakes are injected into the system
Injections are a more sophisticated tool in scammers’ arsenal. In these cases, a biometric sample is modified “on the go.”
The first method involves modifying the signal from the camera, so the data received by the system doesn’t match the actual object presented.
To achieve this, fraudsters position themselves between the physical camera and the biometric verification system. While the camera captures the real scene, the software controlling it can be manipulated—particularly when the image is uploaded via an API.
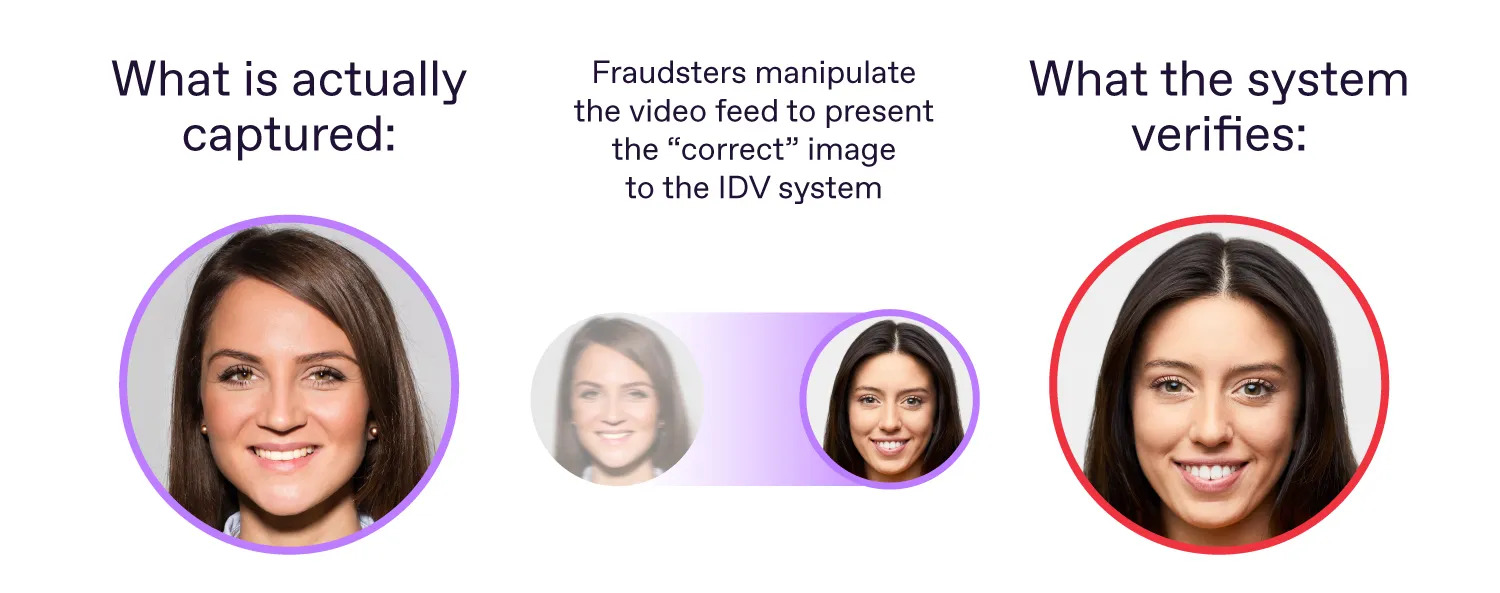
In a signal modification attack, fraudsters trick biometric verification software into seeing an injected image instead of the real one.
Another form of injection attack involves altering a biometric sample during transmission. Here, fraudsters intercept the data as it’s transferred from the capture stage to the analysis stage, modifying it en route.
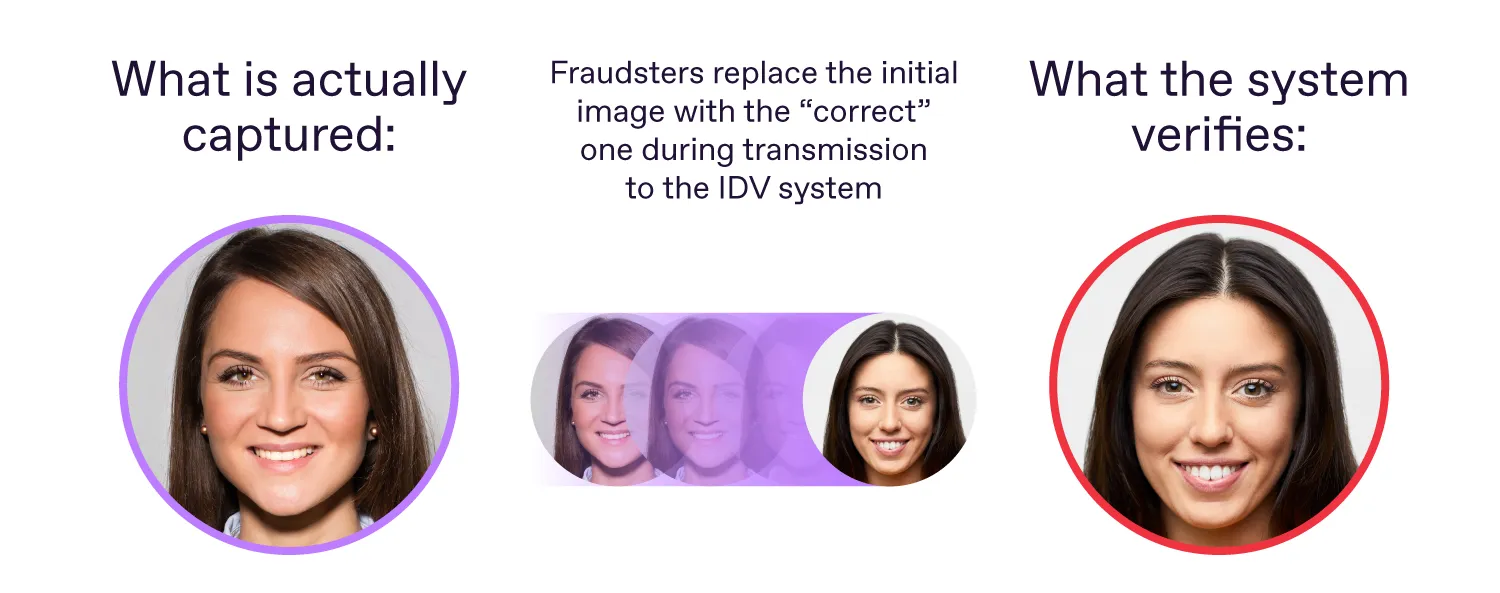
Biometric sample modification enables fraudsters to alter the presented image before it’s analyzed by the software.
As a result, while deepfakes are becoming more advanced and harmful, the methods of exploiting them for bypassing IDV systems remain unchanged. Both presentation and injection attacks rely on the same mechanisms, even with the emergence of AI-generated media.
Does this mean companies already have the tools necessary to tackle the deepfake detection challenge?
Get posts like this in your inbox with the bi-weekly Regula Blog Digest!
The key components of a deepfake detection system
Currently, many businesses rely on an IDV framework that combines ID document verification with biometric checks. According to Regula’s survey, 84% of companies globally use multi-factor authentication and selfie verification to mitigate deepfake-related risks.
Since deepfakes are often presented as on-screen media, advanced features in many IDV solutions, including Regula Face SDK, can detect and reject these attempts. However, more sophisticated attacks, such as deepfake-powered injections, require additional security measures to be revealed.
Let’s explore what tools companies can use to combat these threats.
Liveness detection
Deepfake generation is a resource-intensive process, and many synthetically created images or videos lack perfection. While static AI-generated selfies may look flawless, this illusion often falls apart when live video is required. Deepfake technology struggles to replicate fine details like moles, freckles, or stubble, and can distort accessories such as glasses during movement. This results in inconsistencies in behavior or unnatural artifacts when motion is involved.
For this reason, active liveness detection is essential for a robust deepfake detection system. Randomized activities, such as smiling or turning one’s head, make it harder to generate convincing deepfake videos in real time. Both active and passive liveness detection technologies are also effective at identifying on-screen images.
Mobile-enabled verification
To counter injection attacks, biometric verification systems should ensure data consistency during transmission. Surprisingly, using mobile devices instead of desktop applications provides better protection against injection attempts if the biometric sample source is in the trust perimeter.
Mobile operating systems are generally more secure against invasive actions than PC OSs and web browsers, adding a “default” security layer. However, again, it’s critical to validate the source and/or origin of an image or video.
Another effective measure is the use of hardware-enabled solutions such as self-service kiosks equipped with “trusted” cameras, which prevent deepfakes from leaking into the system.
Secure data transmission
When it comes to identity verification, transferring users' identifiers—such as sensitive personal information and selfies—over secure, encrypted channels is more than a technical requirement. It’s also critical to verify the integrity of the data after transmission.
Encryption ensures that data is protected from interception and tampering, including sophisticated threats like deepfake injections or other advanced fraud attempts. Trusted methods like Public Key Infrastructure (PKI) encryption, tamper-proof protocols, and real-time validation tools help mitigate deepfake risks, as well as more “conventional” threats. These measures align with the use of cryptographic hash functions, where unique data fingerprints are generated and verified to ensure the integrity of transferred data.
Deepfake technology in ID counterfeiting: How to address it?
When it comes to identity documents, there are far fewer AI-generated tools for fraudsters to create them. Nevertheless, this technology is exploited by darknet services like OnlyFake, which can’t be ignored by companies and IDV vendors.
Standard authenticity checks when document layout and the presence of security features are verified are mainly designed for physical ID verification. During online flows, these can be enhanced with an active ID document liveness check, requiring users to tilt the document in front of the camera to demonstrate dynamic security features like holograms or OVI.
Biometric IDs with RFID chips offer an even more secure defense against deepfakes. In regions where electronic identity documents are prevalent, companies can use “biometric passport only” verification to defend against altered or fake IDs. This approach also enhances the customer experience, as NFC verification takes seconds.
So, deepfakes aren’t perfect. Will they be?
AI-generated photos and videos look impressive. While they’re not perfect yet, we have not reached peak AI. Bigger, multimodal models with new capabilities are on the horizon.
However, with the current state of this technology, companies should consider the following inputs when improving their IDV systems:
Deepfakes are on the rise: As the technology becomes more affordable, fraudsters are likely to use synthetic media for attacks more frequently.
Deepfakes are easier to generate than to use: Fraudsters face significant challenges when attempting to bypass IDV systems with deepfakes, thanks to the current verification flow.
Deepfakes are comparable to traditional presentation attacks: Companies that are already adept at detecting on-screen images or masks during selfie verification are well-positioned to counter deepfake attacks. Active liveness detection with randomized challenges remains a reliable tool as well.
Additional security measures are essential: To strengthen defenses, organizations should validate the source of user-submitted data, secure data during transmission, and identify deepfake artifacts and visual inconsistencies.
Regula also doesn't stop advancing its IDV solutions. Learn more about your opportunities with Regula technologies.